Corporate Generative AI Models – Data Consolidation and Access is the Key
- Bill Tolson
- Oct 31, 2023
- 8 min read
Updated: Feb 15, 2024
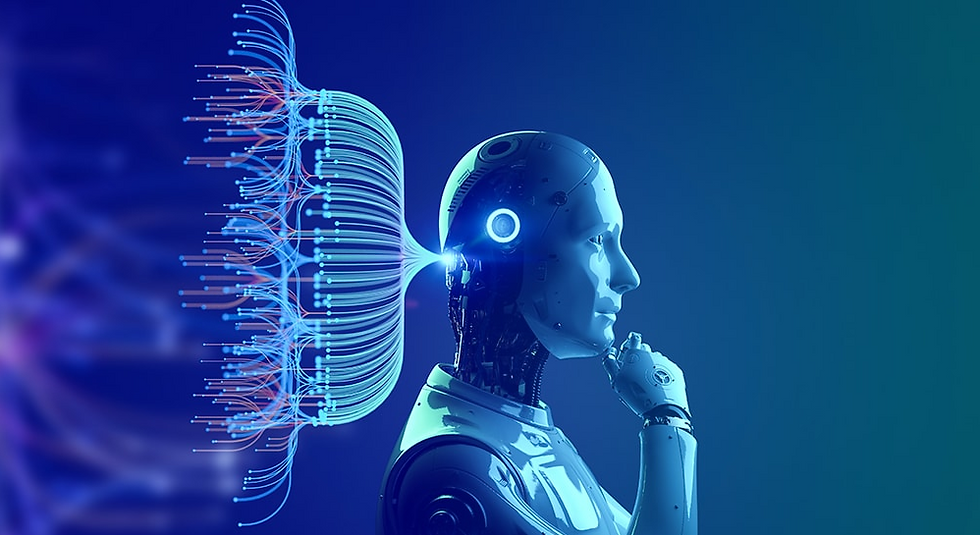
Unmanaged, inaccessible, unstructured data poses a challenge for effective Generative AI model training
Summary: ChatGPT, Google Bard, and various other AI Chat models exploded onto the scene in 2022. These AI models require massive data sets for ongoing training purposes. Now, companies are developing company and industry-specific generative AI models to help them in their particular businesses. Organizations also want to control the sensitive training data they will store in the cloud. As company-specific training data sets are created and grow over time, organizations realize that they must also plan for additional security and accessibility requirements.
This blog will first review what these AI models are (and aren’t.) Then, we will explore the specific storage and accessibility issues associated with large generative AI data training sets. Lastly, I will discuss how restorVault’s storage virtualization solutions can work seamlessly with AI models to solve the various storage, accessibility, and data security challenges.
What are Generative AI models?
Machine Learning (ML) and Artificial Intelligence (AI) can rapidly transform organizations' business operations. AI-powered applications are already used in various industries, from healthcare to retail sales, manufacturing, and our legal system. In fact, ML/AI technology has been used in the legal industry for more than a decade in the form of Predictive Coding. This AI-powered solution can review millions of documents for relevancy in lawsuits at a very high accuracy rate.
However, for ML/AI technology to be effective, it must have access to large amounts of industry and company-specific data for ongoing training. Open AI’s ChatGPT and Google’s BARD AI models are trained on gigantic open-range unstructured data sets scraped from the internet. In fact, ChatGPT was initially trained using 300 billion words taken from books, online texts, Wikipedia articles, and code libraries.
ChatGPT is a conversational artificial intelligence (AI) service based on a Generative Pre-trained Transformer (GPT), a language model built on artificial neural networks developed by OpenAI. It was optimized for human dialogue using Reinforcement Learning with Human Feedback (RLHF). It uses human demonstrations (feedback) and preference comparisons (training cycles) to guide the model toward desired behavior and accuracy.
Companies are adopting customizable corporate AI models, such as the cloud-based ChatGPT Enterprise, to aid them in the industry- and company-specific AI capabilities. However, cloud-based public AI models still have a perceived security issue - sensitive corporate data fed into the AI, such as intellectual property or PII, was not protected from being used as training data for the overall AI platform that others outside your organization could view. In fact, ChatGPT typically collects user inputs (prompts and responses) to enhance and refine the primary ChatGPT model. As a result, confidential information, such as IP and PII, used as examples and training data, becomes part of the AI’s overall training dataset and can be presented to other users outside your organization.
The ChatGPT Enterprise offering has addressed this security issue by ensuring that it does not let the broader ChatGPT model train on your business data or conversations - and the more general models don’t learn from your organization’s usage. Under the ChatGPT Enterprise platform agreement, you own and control your business data.
However, many industries and businesses have unique needs, and cloud-based AI models without contextual data for a particular sector or company cannot provide relevant results.
This is where access to large amounts of potentially sensitive corporate data becomes essential.
Utilizing your company’s data for AI training
Appropriate data must be found and accessed to create the large training data sets needed by the AI platform. Data consolidation is the process of collecting and combining unstructured data from multiple corporate sources into a single, unified, and managed data set for easier access and use. This data can come from application repositories, file shares, cloud repositories, and even local employee-controlled data. Large data consolidation processes can be complex, costly, and time-consuming, but they are essential to realize effective AI capabilities.
However, the other less talked about challenge that companies adopting AI face is the ability of the AI models to quickly and seamlessly access and utilize large amounts of corporate or industry-specific data for ongoing AI training purposes.
Seamless data access is crucial for training AI models. The quality and quantity of data gathered for training AI will determine the effectiveness of the predictive model. Machine learning and artificial intelligence applications would be impossible without high-quality, new, and focused training data. Models would not be able to learn, develop new capabilities, make informed predictions, or extract useful information without the ability to learn from a continuously expanding training data set.
The first task in corporate AI adoption is understanding the types of corporate data that would be useful for AI training and where it currently resides. This is no easy task and will require the company to initiate a data storage repository process to discover where all useful unstructured data is stored and whether it is visible to the enterprise (or stored on employee laptops) or is password-protected.
Data consolidation for AI training
The next step is consolidating the training data sets into a centralized repository for employee and AI model access. Remember that you will be adding to the AI training data regularly (daily) to expand its knowledge, so a technical mechanism will be needed to automatically migrate new data to the AI training repository.
As I have already mentioned, the quality and accuracy of your AI model will be directly proportional to the availability (access) and quality of your training data set. This is not to say that you cannot train the AI model with huge dumps of random data, such as ChatGPT did by scraping the internet for large amounts of random content. However, company or industry-specific data will increase the quality of the output as well as increase the AI response time.
Generally speaking, training an AI model involves feeding it with large amounts of both positive and negative data examples data. It will then “learn” from using human feedback and testing. In each testing cycle, the model is scored against the expected answer to determine the accuracy of the model. Eventually, we want accuracy as close as possible to 100 percent. Effective and timely training is dependent on the AI Model having access to ALL the needed data. That’s where data consolidation comes in.
There are several benefits to data consolidation for AI, including:
· Improved accuracy and reliability:
· Reduced bias:
· Greater insights:
Challenges of data consolidation
Data consolidation can be a complex and challenging process. For peak AI model capability, creating a regularly updated collection of corporate data for ongoing AI training is necessary. This means that access to a wide range of corporate data is needed. Data such as email and attachments, employee work documents, department-specific files, corporate social media activity, customer support traffic, financial records, intellectual property, and many other types of corporate data.
Data consolidation and seamless access are essential considerations for all organizations that plan to incorporate AI models to gain valuable insights from their data. By consolidating data from multiple sources, organizations can improve the accuracy, reliability, and efficiency of their AI feedback, not to mention increased employee productivity.
Where to store training data for ease of AI access and data security
Data consolidation for AI model usage will require an ever-expanding storage resource. The main question is where the AI training data should be stored and managed: on-prem or the cloud.
Storing the growing AI training data set on-prem will drive the need for annual CapEx purchases of additional expensive storage resources. Companies will also need to factor in your backup and DR requirements, which will also require additional enterprise storage resources. Additionally, the AI training data set must be backed up and factored into the company’s disaster recovery processes. These best practice on-prem activities could increase your storage requirements by a factor of 3X, 4X, or more.
Take, for example, the long-held best practice of employing the 3-2-1 backup strategy for data protection. The 3-2-1 backup rule is defined as:
· Having three copies of all protected data
· Stored in two different locations
· One of which is an off-site location such as the cloud
Storage virtualization in the cloud
Cloud storage has proven to be resilient, secure, and easily accessible. Depending on your terms and conditions, adopting cloud storage for a growing training data set makes economic sense. Cloud storage provides dynamic scalability and ensures you never run out of storage for your expanding training data set. Additionally, adopting cloud storage could reduce your training data set storage requirements. No longer would the necessity to create three backup copies (3-2-1) of your data as a data protection strategy be needed. Additionally, the DR storage resources could also be reduced.
The primary storage virtualization strategy for AI training data sets involves utilizing the AI platform’s cloud storage capability. In this scenario, your specific training data set stored within the AI platform could be virtualized to another (more secure) cloud platform. This would ensure that your sensitive training data stored in the AI platform would be replaced with live virtual pointers, directing the AI model to access the training data stored on the third-party cloud. With this storage strategy, most (or all) of your sensitive corporate training data could be inaccessible to other organizations using the AI platform. This storage strategy could also reduce your overall costs and provide more data protection against ransomware.
Why storage virtualization for AI training data set storage?
Your AI and associated training data will collect, store, and use a huge amount of your organization’s sensitive data. In reality, these training data sets will become a major target for cyber thieves and ransomware because of the sensitivity of the data stored. Because of the increased focus on these valuable data sets, ensuring the training data is secure and protected should be a primary focus of data management and security.
Storage virtualization adds an extra level of data security while reducing overall storage costs by reducing the number of copies for backup and DR.
restorVault’s storage virtualization solutions for on-prem and cloud storage
restorVault’s cloud storage virtualization solution stores the AI training data in the highly secure restorVault cloud. Additionally, it creates and stores a complete second full copy, encrypted and stored on immutable storage. Additionally, the restorVault cloud solution will not require the data set to be backed up using the 3-2-1 backup process or need to generate additional DR copies.
restorVault has been a leading innovator of storage virtualization solutions for several years. restorVault’s patented Copy Data Virtualization (CDV) and Offload Data Virtualization solutions allow enterprise users to migrate data automatically (based on policies) to lower-cost and more secure cloud repositories while keeping fast and seamless access to the migrated files.
restorVault’s CDV solution accomplishes this by migrating the existing large file sets into restorVault’s secure and immutable Compliant Cloud Archive (CCA), where all data is encrypted and stored on immutable storage tiers for the highest level of data security from ransomware attacks.
You can then incorporate an on-prem or cloud-based file server to store active data as well as the migrated data’s pointers. In this way, the AI can see and use the virtualized data.
The restorVault Compliant Cloud Archive (CCA) stores two copies of the migrated training data. All files stored in CCA are encrypted and stored on the immutable storage tier in the restorVault cloud in case of a ransomware attack. In case of data corruption of ransomware attack that affects the on-prem file server, the file pointers can be pushed down to the target file system server in minutes, ensuring continued access to the data for the employees as well as the AI model.
restorVault’s patented Copy Data Virtualization and Offload Data Virtualization solutions provide a refined and trusted solution to safely store your most sensitive unstructured data safely and inexpensively in a trusted and immutable cloud vault while presenting a consolidated view of the data for easy access by the AI.
Please get in touch with us today to learn how restorVault can help your company save money while increasing data security and storage capacity.
댓글